2018.03.01.14
Files > Volume 3 > Vol 3 No 1 2018
NOTICIAS Y OPINIONES / NEWS AND VIEWS
Current methods of breast cancer diagnosis
Métodos actuales en el diagnóstico del cáncer de mama
Keila Gómez, Juan Carlos Laglaguano, Raúl Rodríguez
Available from: http://dx.doi.org/10.21931/RB/2018.03.01.14
ABSTRACT
Breast cancer is the most frequent cancer among women, and represents the second highest number of deaths around the world, it’s difficult to diagnose because there are different types of breast cancer. The new methods for the detection of breast cancer are very promising because they guarantee that the patients received the specific kind of treatment. At present, there are breast cancer detection techniques which are very expensive, traditional and complicated, which leads us to the need to develop easy-to-use and more sensitive devices. In this review, we will analyze more recent methods of breast cancer diagnosis such as molecular signatures, micro-RNA, and bioinformatic methods.
Keywords: Breast cancer, diagnostic methods
RESUMEN
El cáncer de mama es el más frecuente entre las mujeres y representa el segundo mayor número de muertes en todo el mundo, es difícil ser diagnosticado porque hay diferentes tipos de cáncer de mama. Los nuevos métodos para la detección de cáncer de mama son muy prometedores porque garantiza que los pacientes recibieron el tipo adecuado de tratamiento. En la actualidad, existen técnicas de detección de cáncer de mama, que son muy costosas, tradicionales y complicadas, lo que nos lleva a la necesidad de desarrollar dispositivos más fáciles de usar y más sensibles. En esta revisión, analizaremos los métodos de diagnóstico de cáncer de mama más recientemente, como las firmas moleculares, el microARN y los métodos bioinformáticos.
Palabras claves: Cáncer de mama, métodos diagnósticos,
INTRODUCTION
Breast cancer is the most common cancer diagnosed in women around the world; Ecuador is not an exception (Tab. 1). Cancer starts when breast cells begin to grow in an uncontrolled manner way. To understand breast cancer, we must know the basic anatomical structure of the breast. Each breast is composed of lobules, lobes, and bulbs that are connected by ducts. Lobs are divided into smaller structures called lobules that end in tiny bulbs that can produce milk. Breast cancer is classified based on the anatomical structure and according to this classification, the most common types of breast cancer are the ductal carcinoma and invasive lobular carcinoma.1Differentiating all breast tumor types is relevant in the clinical treatment case to guarantee that patients received the most appropriate kind of therapy.2 Currently, there are breast cancer detection techniques, which are very expensive, traditional and complicated, which leads us to the need to develop innovative, determined and ultrasensitive devices.3 In this review, we will conduct a study on the new methods of breast cancer diagnosis currently in use such as the use of molecular signatures, biosensors, micro RNA, and bioinformatics methods .
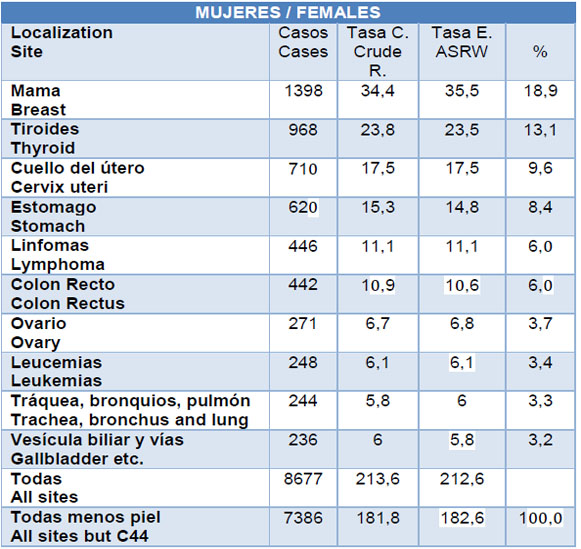
Table 1: (“Epidemiología del Cáncer en Quito 2006-2010 by SOLCA Quito - issuu,” n.d.). Available from:https://issuu.com/solcaquito/docs/epidemiologia_de_cancer_en_quito_20
Molecular signatures
The acceptance of molecular profiles of DNA, RNA, and proteins reveals significant differences in fundamental biology, and this begins to have an impact on patients who are handling this technique. Diverse bioinformatics devices have been refined using DNA or RNA-based signatures to classify the illness into biologically and clinically essential subgroups.2 A characterization of cancer will obtain somatic mutations in the tumor genome, which include base substitutions, insertions, and deletions, changes in the number of copies and structural rearrangements. Nowadays, some mutations accumulate in the cell lineage, and individual or collective work gives a significant selective advantage to the tumor cell. Its form of distribution serves as a trace to discover underlying mutational processes that aid the development of the tumor.4,5 A characterization of cancer is that it obtains somatic mutations in the tumor genome, which includes substitutions of bases, insertions, and deletions, changes in the number of copies and structural rearrangements.6 A large number of classifiers are found which based on the number of copies for breast cancer.7 These classifiers can be the use of random forest variants,8logistic regression and group loop,9 fused support vector machine10 and the union of functions that have supervision or not which use silhouette techniques to estimate clusters.11 In the supervised as in the unsupervised FC, excellent performance found when ordering the CGH data of the tumor samples, due to the ability to exclude the unwanted correlation bias.7 Also, to have an idea of the etiology of the tumor, substitution signatures and patterns of structural rearrangements give a more definite vision. A large number of studies of complete genomes of breast cancer12 defined six reconditioning firms which were based on type and size of disposition, and also in the extension of the alteration in the whole genome (grouped or dispersed).2
Biomarkers
In recent years, research in the field of molecular biology has contributed to the improvement in the diagnosis of cancer. Tumor markers are biochemical indicators when a tumor is present; this incorporates cell surface antigens, cytoplasmic proteins, enzymes, and hormones. In clinical practice, the term is used to refer to molecules that are available in plasma, body fluids, solid tumors, circulating tumor cells, lymph nodes and bone marrow.13 The technique of immunohistochemical detection of specific antigens of the cell cycle is used to evaluate the proliferative activity of cells.14
For example, Ki67 is located in the cell nucleus where it only binds to the perichromosomal layer in cells that divide and grow actively; this is used as a marker to determine cell proliferation. In consequence, changes in Ki67 expression in growing tumor cells compared to healthy cells can be used as an early predictor for treatment efficiency and can also be used as a prognostic factor for long-term outcomes in cancer patients.15
Salivary biomarkers are being used to characterize breast cancer, correlating total salivary sialic acid and breast cancer. The sialic acid found in the final residues of the carbohydrate chains are biologically necessary and essential for the functioning of the glycoconjugates. In patients who have cancer, these markers are altered. The increase of sialylation of the glycoconjugates of the cell surface is localized between the critical molecular changes associated with malignant transformation and cancer progression.16 Studies have found higher levels of salivary sialic acid in patients who have breast cancer compared to the healthy control group.17 Finally, the future of tumor biomarkers is promising, because they provide information on the biological behavior of the tumor and this is of great importance because they reduce the mortality of patients who have cancer.
Micro-RNA
MicroRNA are small noncoding RNA molecules around of 18-25 nucleotides in length.18 They regulate gene expression to repress or/and promote mRNA degradation.19 miRNAs can control multiple critical pathways that are involved in physiological as well as pathological processes. The downregulation of miRNA genes could result from aberrant hypermethylation, as in the case of miR-9-1 gene in breast cancer,20 or from histone deacetylation and tri-methylation, as in the case of miR-29 in B-cell lymphomas.21 MiRNA is present in several biological fluids, among them breast milk.22
Breast cancer could be classified according to different parameters such as histology, immunopathology, mRNA expression profiling, and miRNA expression signature.23 MiRNA signature can sub-classify breast cancer,24 and can even determine new subtypes, as recently reported.25 MiRNA is expressed in breast cancer, as manifest by microarray profiling of tumor and normal breast tissues.26 Various studies reveal that diverse functions of the dysregulated miRNA in malignant breast transformation whereby they act as oncogenes (oncomiRNA) or as tumor suppressors. In breast cancer, miRNA has been shown to regulate cell cycle progression, apoptosis, angiogenesis, epithelial-mesenchymal transition, tumor microenvironment, migration, invasion, metastasis and drug resistance, as well as the differentiation and self-renewal of breast cancer stem cells.27 For strength to endocrine treatments, the serum miRNA has the potential to serve as a biomarker of EBC.
Diagnostic methods of microRNA in breast cancer
Studies carried out had shown that the miR-21 that is the most researched onco miRNAs in this malignant disease serves as a diagnostic biomarker for EBC.28–34 The studies detected miR-21 in breast tissues using experimental technology as microarray/sequencing/RT-qPCR followed by validation in serum and plasma.33–35Other studies were carried out in array panels on plasma samples followed by verification in plasma, and the technique that implemented was RT-qPCR32 or started with RT-qPCR on tissues then direct serum RT-qPCR.28Signally, serum miR-21 exhibited higher sensitivity in EBC diagnosis than other conventional cancer markers, such as CA153 and CEA.30 In another study with the same method, the miR-155 shown to upregulated in serum and tissues of breast cancer patients.26,36–39
MiR-12b and miR-145 were the first to be downregulated and miR-21 to be upregulated in BC tumors compared to normal breast tissues,40–42 had used in ERα (estrogen receptor alpha) + BC diagnostic the MammaPrint, Oncotype DX and PAM50 gene arrays. One study of miRNA expression with current scores from Oncotype DX on 23 human BC tumors reported that in left of 7 family members reduced expression with high RS and high expression of miR-377-5p, miR-663b and miR 3648 associated with high RS scores.
Prognostic miRNAs for tumor versus regular class prediction were determined by using the tool support vector machine and prediction analysis of software microarrays, these algorithms used too for cross-validation. Another method of diagnostic is the Northern blotting analysis that were done in RNA sample (10 mg each) were electrophoresed on 15% acrylamide, 7 mol/L urea criterion precast gels and transferred onto Hybond-N+ membrane.39 The Hybridization was done at a temperature of 37° C in 7 % SDS/0.2 mol/L Na2PO4 (pH 7.0) for a time of sixteen hours. The membranes were washed at 42°C twice with two standard saline phosphate one mmol/L EDTA, and 0.1% SDS and again twice with 0.5 SSPE/0.1% SDS. The oligonucleotides used as probes are the complementary sequences of the mature miRNA, used to normalize expression levels. In the results the microarray analysis they carried out the study of the Northern blot of the differentially expressed miRNAs.26They analyzed the expression of miR-125b, miR-145, and miR-21 both in human breast cancers and in breast cancer cell line. Moreover, all Northern blots confirmed results obtained by the microarray analysis. The miRNAs: miR-10b, miR125b, miR-145, miR-21, and miR-155 were analyzed using three algorithms, miRanda, TargetScan, and PicTar, that are commonly used to predict human miRNA gene target.
Bioinformatic methods
There are many data about breast cancer such as medical records, clinical data or biomedical images are collected and available on databases. Some of the famous databases about breast cancer are the Wisconsin Breast Cancer Database and ArrayExpress. Wisconsin Breast Cancer Database is renowned because it offers information of computed features of the breast cancer cells nuclei.43 From digitized images, Wisconsin Breast Cancer Database offers an evaluation 32 features such as radius, texture, perimeter, area, smoothness, compactness, concavity, hollow points, symmetry fractal dimension and diagnosis (malignant or benignant) from digitized images of a fine-needle aspirate of a breast mass.44 Another database is Array Express, that is a public repository for microarray data. Their databases not only contain data about cancer but also from functional genomics experiments of thousands of clinical studies, and provides these data for the reuse to the research community.45
Despite the existence of available data about breast cancer, there are no suitable methods to analyze the significant amounts of data. It will be impossible for humans to process vast amounts of information so that computers have been fundamental for the development of science in general. Bioinformatics combines computer sciences, biological sciences, mathematics and engineering to process and interpret data. One of the most used fields of bioinformatics is artificial intelligence. In simple terms, artificial intelligence it would be defined as the use of a computer to simulate human intelligence and ability to learn.46 Data mining and machine learning are two related artificial intelligence technologies; they can be related to each other. Machine Learning refers to the study, design, and development of the algorithms that give computers the capability to learn without being explicitly programmed.47 Data Mining is the process that starting from apparently unstructured data tries to extract knowledge and interesting unknown patterns. During this process, the use of machine learning techniques is necessary for most of the data mining techniques.
Data mining is the automated or convenient extraction of information patterns from data stored in massive information repositories, or data streams.47 The primary medical applications of data mining are patient phenotype cohorts,48 pharmacovigilance,49 clinical pathways,50 healthcare process,51 disease progression,52 and deep learning for precision medicine and Human-computer interaction and knowledge discovery in dataset approach in biomedical informatics. Data mining techniques are based on training and testing the system regarding the characteristic or feature that have to be analyzed. The complexity of the data mining process depends on how extensive are the data to be examined and the number of elements to integrate into the mining process.
In the data mining is a multistep process.53 The first step implies the collection, preprocessing, and normalization of data. Data is collected from heterogeneous sources and converted into homogenous. The second step is training and testing of the algorithms to obtain some meaningful information in an automatized way. The third step analyzes the processed data and represents it in a standardized format. Finally, the upshots of data mining progression are useful in the prediction, of for example, the presence or not of cancer breast cells or the type of breast cancer by the use of biomedical images54 or analyzing DNA microarrays.55 In general, data mining is used to test a hypothesis or to discover some new or hidden patterns53. BioInformatic methods are fundamental to increase the accuracy of breast cancer diagnosis starting with clinical data.
CONCLUSION
New methods of cancer diagnosis have the potential to improve the accuracy of diagnosis and avoidance invasive techniques that are traumatic for the patients.
REFERENCES
1. Weigelt B, Geyer FC, Reis-Filho JS. Histological types of breast cancer: How special are they? Mol Oncol. 2010 Jun 1;4(3):192–208.
2. Lal S, McCart Reed AE, de Luca XM, Simpson PT. Corrigendum to “Molecular signatures in breast cancer” [Methods 131 (2017) 135–146]. Methods [Internet]. [cited 2017 Nov 1]; Available from: https://www.sciencedirect.com/science/article/pii/S1046202318300227
3. Mittal S, Kaur H, Gautam N, Mantha AK. Biosensors for breast cancer diagnosis: A review of bioreceptors, biotransducers and signal amplification strategies. Biosens Bioelectron. 2017 Feb 15;88:217–31.
4. Alexandrov LB, Nik-Zainal S, Wedge DC, Aparicio SAJR, Behjati S, Biankin AV, et al. Signatures of mutational processes in human cancer. Nature. 2013 Aug 22;500(7463):415–21.
5. Nik-Zainal S, Alexandrov LB, Wedge DC, Van Loo P, Greenman CD, Raine K, et al. Mutational processes molding the genomes of 21 breast cancers. Cell. 2012 May 25;149(5):979–93.
6. Hicks J, Krasnitz A, Lakshmi B, Navin NE, Riggs M, Leibu E, et al. Novel patterns of genome rearrangement and their association with survival in breast cancer. Genome Res. 2006 Dec;16(12):1465–79.
7. Tolosi L, Lengauer T. Classification with correlated features: unreliability of feature ranking and solutions. Bioinforma Oxf Engl. 2011 Jul 15;27(14):1986–94.
8. Breiman L. Random Forests. Mach Learn. 2001 Oct 1;45(1):5–32.
9. Meier L, van de Geer S, Bühlmann P. The group lasso for logistic regression. J R Stat Soc Ser B. 2008;70(1):53–71.
10. Rapaport F, Barillot E, Vert J-P. Classification of arrayCGH data using fused SVM. Bioinformatics. 2008 Jul 1;24(13):i375–82.
11. Park MY, Hastie T, Tibshirani R. Averaged gene expressions for regression. Biostatistics. 2007 Apr 1;8(2):212–27.
12. Nik-Zainal S, Davies H, Staaf J, Ramakrishna M, Glodzik D, Zou X, et al. Landscape of somatic mutations in 560 breast cancer whole-genome sequences. Nature. 2016 02;534(7605):47–54.
13. Stearns V, Yamauchi H, Hayes DF. Circulating tumor markers in breast cancer: accepted utilities and novel prospects. Breast Cancer Res Treat. 1998;52(1–3):239–59.
14. Juríková M, Danihel Ľ, Polák Š, Varga I. Ki67, PCNA, and MCM proteins: Markers of proliferation in the diagnosis of breast cancer. Acta Histochem. 2016 Jun;118(5):544–52.
15. Dowsett M, Nielsen TO, A’Hern R, Bartlett J, Coombes RC, Cuzick J, et al. Assessment of Ki67 in breast cancer: recommendations from the International Ki67 in Breast Cancer working group. J Natl Cancer Inst. 2011 Nov 16;103(22):1656–64.
16. Shah MH, Telang SD, Shah PM, Patel PS. Tissue and serum alpha 2-3- and alpha 2-6-linkage specific sialylation changes in oral carcinogenesis. Glycoconj J. 2008 Apr;25(3):279–90.
17. Oztürk LK, Emekli-Alturfan E, Kaşikci E, Demir G, Yarat A. Salivary total sialic acid levels increase in breast cancer patients: a preliminary study. Med Chem Shariqah United Arab Emir. 2011 Sep;7(5):443–7.
18. Lee JA, Lee HY, Lee ES, Kim I, Bae JW. Prognostic Implications of MicroRNA-21 Overexpression in Invasive Ductal Carcinomas of the Breast. J Breast Cancer. 2011 Dec;14(4):269–75.
19. Role of Increased Mir-29b-1 and Mir-29a in Endocrine-Resistant Breast Cancer : Metastasis and Tumor Progression, Neoplasia of Endocrine Tissue, Therapies for Cancer, and Tumorigenesis (posters). ENDO Meet [Internet]. [cited 2017 Nov 14]; Available from: http://press.endocrine.org/doi/abs/10.1210/endo-meetings.2016.TB.7.SUN-096
20. Epigenetic inactivation of microRNA gene hsa-mir-9-1 in human breast cancer. - PubMed - NCBI [Internet]. [cited 2017 Nov 14]. Available from: https://www.ncbi.nlm.nih.gov/pubmed/17948228
21. Chen X, Liang H, Zhang J, Zen K, Zhang C-Y. Secreted microRNAs: a new form of intercellular communication. Trends Cell Biol. 2012 Mar;22(3):125–32.
22. Hanke M, Hoefig K, Merz H, Feller AC, Kausch I, Jocham D, et al. A robust methodology to study urine microRNA as tumor marker: microRNA-126 and microRNA-182 are related to urinary bladder cancer. Urol Oncol. 2010 Dec;28(6):655–61.
23. Bertos NR, Park M. Breast cancer — one term, many entities? J Clin Invest. 2011 Oct 3;121(10):3789–96.
24. Blenkiron C, Goldstein LD, Thorne NP, Spiteri I, Chin S-F, Dunning MJ, et al. MicroRNA expression profiling of human breast cancer identifies new markers of tumor subtype. Genome Biol. 2007;8(10):R214.
25. Bhattacharyya M, Nath J, Bandyopadhyay S. MicroRNA signatures highlight new breast cancer subtypes.Gene. 2015 Feb 10;556(2):192–8.
26. Iorio MV, Ferracin M, Liu C-G, Veronese A, Spizzo R, Sabbioni S, et al. MicroRNA gene expression deregulation in human breast cancer. Cancer Res. 2005 Aug 15;65(16):7065–70.
27. Li L, Xiao B, Tong H, Xie F, Zhang Z, Xiao GG. Regulation of breast cancer tumorigenesis and metastasis by miRNAs. Expert Rev Proteomics. 2012 Dec;9(6):615–25.
28. Asaga S, Kuo C, Nguyen T, Terpenning M, Giuliano AE, Hoon DSB. Direct serum assay for microRNA-21 concentrations in early and advanced breast cancer. Clin Chem. 2011 Jan;57(1):84–91.
29. Chen H, Liu H, Zou H, Chen R, Dou Y, Sheng S, et al. Evaluation of Plasma miR-21 and miR-152 as Diagnostic Biomarkers for Common Types of Human Cancers. J Cancer. 2016;7(5):490–9.
30. Gao J, Zhang Q, Xu J, Guo L, Li X. Clinical significance of serum miR-21 in breast cancer compared with CA153 and CEA. Chin J Cancer Res. 2013 Dec;25(6):743–8.
31. Matamala N, Vargas MT, González-Cámpora R, Miñambres R, Arias JI, Menéndez P, et al. Tumor microRNA expression profiling identifies circulating microRNAs for early breast cancer detection. Clin Chem. 2015 Aug;61(8):1098–106.
32. Ng EKO, Li R, Shin VY, Jin HC, Leung CPH, Ma ESK, et al. Circulating microRNAs as specific biomarkers for breast cancer detection. PloS One. 2013;8(1):e53141.
33. Si H, Sun X, Chen Y, Cao Y, Chen S, Wang H, et al. Circulating microRNA-92a and microRNA-21 as novel minimally invasive biomarkers for primary breast cancer. J Cancer Res Clin Oncol. 2013 Feb;139(2):223–9.
34. Wu Q, Lu Z, Li H, Lu J, Guo L, Ge Q. Next-generation sequencing of microRNAs for breast cancer detection. J Biomed Biotechnol. 2011;2011:597145.
35. Mar-Aguilar F, Mendoza-Ramírez JA, Malagón-Santiago I, Espino-Silva PK, Santuario-Facio SK, Ruiz-Flores P, et al. Serum circulating microRNA profiling for identification of potential breast cancer biomarkers. Dis Markers. 2013;34(3):163–9.
36. Shaker O, Maher M, Nassar Y, Morcos G, Gad Z. Role of microRNAs -29b-2, -155, -197 and -205 as diagnostic biomarkers in serum of breast cancer females. Gene. 2015 Apr 10;560(1):77–82.
37. Sochor M, Basova P, Pesta M, Dusilkova N, Bartos J, Burda P, et al. Oncogenic microRNAs: miR-155, miR-19a, miR-181b, and miR-24 enable monitoring of early breast cancer in serum. BMC Cancer. 2014 Jun 18;14:448.
38. Sun Y, Wang M, Lin G, Sun S, Li X, Qi J, et al. Serum microRNA-155 as a potential biomarker to track disease in breast cancer. PloS One. 2012;7(10):e47003.
39. Győrffy B, Hatzis C, Sanft T, Hofstatter E, Aktas B, Pusztai L. Multigene prognostic tests in breast cancer: past, present, future. Breast Cancer Res BCR [Internet]. 2015 [cited 2017 Nov25];17(1). Available from: https://www.ncbi.nlm.nih.gov/pmc/articles/PMC4307898/
40. Kittaneh M, Montero AJ, Glück S. Molecular Profiling for Breast Cancer: A Comprehensive Review. Biomark Cancer. 2013 Oct 29;5:61–70.
41. Wen HY, Krystel-Whittemore M, Patil S, Pareja F, Bowser ZL, Dickler MN, et al. Breast carcinoma with an Oncotype Dx recurrence score <18: Rate of distant metastases in a large series with clinical follow-up. Cancer. 2017 Jan 1;123(1):131–7.
42. Tibshirani R, Hastie T, Narasimhan B, Chu G. Diagnosis of multiple cancer types by shrunken centroids of gene expression. Proc Natl Acad Sci U S A. 2002 May 14;99(10):6567–72.
43. Furey TS, Cristianini N, Duffy N, Bednarski DW, Schummer M, Haussler D. Support vector machine classification and validation of cancer tissue samples using microarray expression data. Bioinforma Oxf Engl. 2000 Oct;16(10):906–14.
44. UCI Machine Learning Repository: Breast Cancer Wisconsin (Diagnostic) Data Set [Internet]. [cited 2017 Nov 27]. Available from: https://archive.ics.uci.edu/ml/datasets/Breast+Cancer+Wisconsin+(Diagnostic)
45. ArrayExpress < EMBL-EBI [Internet]. [cited 2017 Nov 27]. Available from: https://www.ebi.ac.uk/arrayexpress/
46. Hamet P, Tremblay J. Artificial intelligence in medicine. Metabolism. 2017 Apr;69S:S36–40.
47. Samuel AL. Some studies in machine learning using the game of Checkers. Ibm J Res Dev. 1959;71–105.
48. Shivade C, Raghavan P, Fosler-Lussier E, Embi PJ, Elhadad N, Johnson SB, et al. A review of approaches to identifying patient phenotype cohorts using electronic health records. J Am Med Inform Assoc JAMIA. 2014 Mar;21(2):221–30.
49. White RW, Tatonetti NP, Shah NH, Altman RB, Horvitz E. Web-scale pharmacovigilance: listening to signals from the crowd. J Am Med Inform Assoc JAMIA. 2013;20(3):404–8.
50. Kinsman L, Rotter T, James E, Snow P, Willis J. What is a clinical pathway? Development of a definition to inform the debate. BMC Med. 2010 May 27;8:31.
51. Mans RS, Aalst WMP van der, Vanwersch RJB. Process Mining in Healthcare: Evaluating and Exploiting Operational Healthcare Processes [Internet]. Springer International Publishing; 2015 [cited 2018 Feb 10]. (SpringerBriefs in Business Process Management). Available from: //www.springer.com/gb/book/9783319160702
52. Mould DR. Models for disease progression: new approaches and uses. Clin Pharmacol Ther. 2012 Jul;92(1):125–31.
53. Data Mining: Concepts and Techniques - 3rd Edition [Internet]. [cited 2017 Nov 28]. Available from: https://www.elsevier.com/books/data-mining-concepts-and-techniques/han/978-0-12-381479-1
54. Han Z, Wei B, Zheng Y, Yin Y, Li K, Li S. Breast Cancer Multi-classification from Histopathological Images with Structured Deep Learning Model. Sci Rep. 2017 Jun 23;7(1):4172.
55. Houssami N, Lee CI, Buist DSM, Tao D. Artificial intelligence for breast cancer screening: Opportunity or hype? Breast Edinb Scotl. 2017 Dec;36:31–3.
Received: 18 november 2017
Approved: 20 january 2018
1Universidad de Investigación de Tecnología Experimental Yachay Tech
Juan Carlos Laglaguano
https://orcid.org/0000-0003-0740-7113
Raúl Heriberto Rodríguez E.
https://orcid.org/0000-0003-3281-2700
Keila Franchesca Gomez Acosta
Email: [email protected]
https://orcid.org/0000-0002-9979-2671