2023.08.01.66
Files > Volume 8 > Vol 8 No 1 2023

1 Department of Agricultural Machines and Equipment, College of Agricultural Engineering Sciences
University of Baghdad, Iraq; [email protected].
2 Department of Agricultural Machines and Equipment, College of Agricultural Engineering Sciences
University of Baghdad, Iraq;
*Correspondinh author. [email protected].
Available from: http://dx.doi.org/10.21931/RB/2023.08.01.66
ABSTRACT
This study was conducted in a laboratory experiment at the University of Baghdad, College of Science, computing Department, 5 km from the center of Baghdad city, in 2021 to evaluate the sorting method for the tomato crop. The experiments were conducted in a factorial experiment under a complete randomized design with three replications and using SAS analysis, artificial neural network, image processing, the study of external characteristics, and physical features; fruit surface area and fruit circumference were 1334.46 cm2,57.53 cm2 and free diseases. The error value was less than zero, while training with outputs recorded the highest value and which was 5. The neural network's performance between the input and the mean square of the regression, as recorded as the best value to validate the performance, was 58.11 in the second period. The importance of fruit circumference is attributed to the sorting and grading of fruits, especially in packing boxes and marketing.
Keywords: Automated grading and sorting, traditional grading and sorting, image processing, computer vision, Artificial neural networks
INTRODUCTION
The scientific name of tomato is Solanum lycopersicum is considered one of the important crops and is grown over long distances in Iraq; its cultivation is concentrated in Basra, Najaf and Babylon. Horticultural and fruit crops are among the essential crops to achieve food security and stability to satisfy the growing population. . In traditional sorting, workers sort the fruits manually according to the required fruit size.
One of the disadvantages of this process is the high costs due to fruit spoilage and high fruit costs. The different sorting of the same fruit for foreign workers affects sorting accuracy, as well as any excess force or error in holding the fruit can damage and rot the fruit and cause loss of money and product.
In contrast, automatic sorting can be used in different ways depending on the vision of the machine that employs a camera with sensors and image processing with special programs that use computer sorting. Grading based on color, shape, size, maturity, mottling, quality and freedom from disease, and the accuracy rate is 69-75% and up to 94-98% depending on the type of fruit 1. 1. Computer imaging is also characterized by transmitting information, translating it and making decisions. It is done by taking pictures and translating the digital badges on the computer. Artificial neural networks are known to be inspired by the functions of the human brain, simulating complex operations such as pattern generation, perception, learning, and decision-making. The human brain is made up of billions of neurons that communicate and process any information that is presented. Similarly, the simplified model of the structure of a biological neural network consists of interconnected processing units organized into a specific topology 2. Vegetable and fruit crops are essential for food security and stability to meet the increasing population. The most important fruit crops are the tomato Solanum lycopersicum 3; most of its species are endemic to the regions of Ecuador, Peru, Bolivia and Chile, as these species grow in different environments, from the dry environment to the humid climate, as well as they are sometimes grown in greenhouses in cold weather. And this varying environmental diversity led to a variety in the plant's morphological, physiological and molecular forms 4,5. Tomato is consumed in various ways, including raw tomatoes, sauces, salads, and drinks, a fruit rich in lycopene, which has beneficial health effects for humans 6. Tomatoes are widely grown in Iraq in various forms of cultivation, but productivity is still low 7. According to the scientific research method, this study aims to the effect of traditional and Automated Sorting on Some Tomatoes Properties.
MATERIALS AND METHODS
The experiment was conducted to check the sorting and grading of crops using image processing technology within the image processing laboratory. College of Science \ Technology Department at the \ University of Baghdad, employing a locally factory-produced studio cube-shaped with dimensions 75 * 75 * 50 shown in figure 1 and a camera with a sensor of the coupling device, and also the studio was equipped with RGB lighting (red - green - blue), and also the pictures were taken within the studio to review the external characteristics of the fruits that are diseases free using image processing technology and therefore the artificial neural network, still as a study of the physical characteristics of the fruits, fruit circumference, fruit surface area. Statistical analysis by using SAS analysis, the factorial experiment under the whole random design system with a probability of 0.05. Tomatoes and five replications, three pictures of the identical fruit were taken from the highest, bottom and side, and the total number of images taken within the experiment is 64. The RGB (red-green-blue) is placed inside the studio because the camera is placed 15 cm removed from the fruits to make the image prominent and of high resolution. The fruits were manually replaced one by one. Simultaneous analysis of the fruits was conducted to review the external characteristics of the fruit and disease free. An artificial neural network with 20 nodes, a training group of 70%, verification of 15%, and testing of 15%, so a study was conducted for the physical characteristics of the fruits, including the fruit circumference and surface.
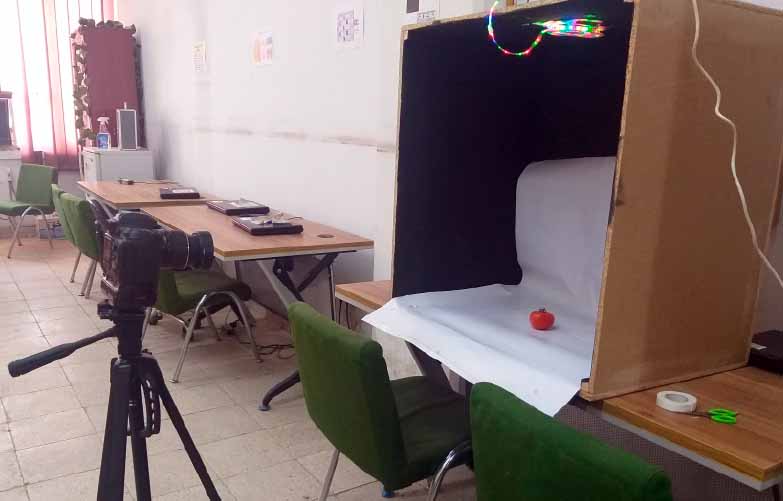
Figure 1. Studio for photos, Tomatoes and five replications, three pictures of the identical fruit were taken from the highest, bottom and side
RESULTS AND DISCUSSION
Fruit surface area
Table (1) shows the effect of the sorting method on some characteristics of the tomato plant (color, shape and size) and its interaction with the surface area of the fruit by using the SAS analysis program. The results showed the highest value for the size characteristic, followed by form than color. Recorded results were 1336.65, 1334.67 and 1332.06 cm2, respectively, with a rate of 1334.46 cm2, due to sorting and grading based on surface area associated with the plant, color and shape of the size of the fruit. This, in turn, depends on the fruit type, shape and color. This is consistent with Arjenaki et al. 2013.1
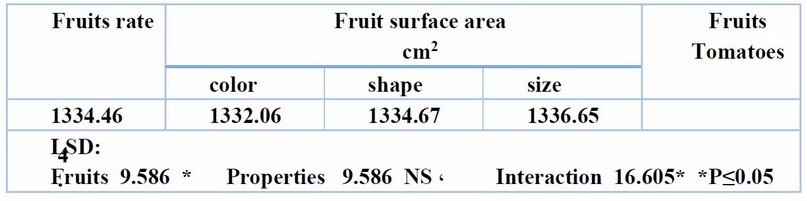
Table 1. Effect of sorting method and properties on Fruit surface area cm2
Table (1) shows the effect of the sorting method on some characteristics of the tomato plant (color, shape and size) and its interaction with fruit circumference of the fruit using the SAS analysis program. The results showed the highest value for the size characteristic, followed by shape, color, and recorded results were 57.53 cm2. The importance of fruit circumference is attributed to the sorting and grading of fruits, especially in packing boxes and marketing 8.
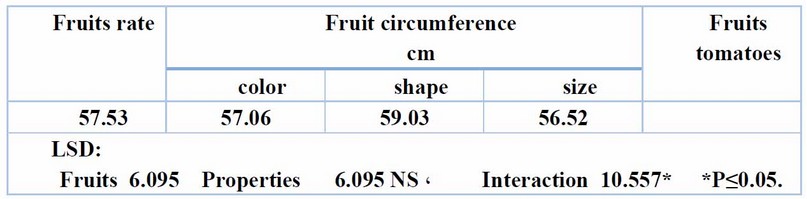
Table 2. Effect of sorting method and properties on fruit circumference
Diseases free
The neural network's performance between the input and the mean square of the regression, as it recorded the best value to validate the performance, was 58.11 in the second period. The performance value of the network is tremendous. Therefore the test samples did not affect the training and thus provided an independent measure of the network's performance during and after the movement, as shown in figure 2 A 7. Proportional values for training inputs and outputs, verifying inputs and outputs, and testing inputs and outputs, error and proportionality, as it showed that the error value was less than zero. In contrast, training with outcomes recorded the highest value of 5. The figure notes the loss values with time during training, as the loss values are calculated across a subset of the data. If a value decreases with the validation value, then this means that the time is appropriate to stop the training because after that, it is not appropriate, and this is called early stopping, as shown in figure 2 B.
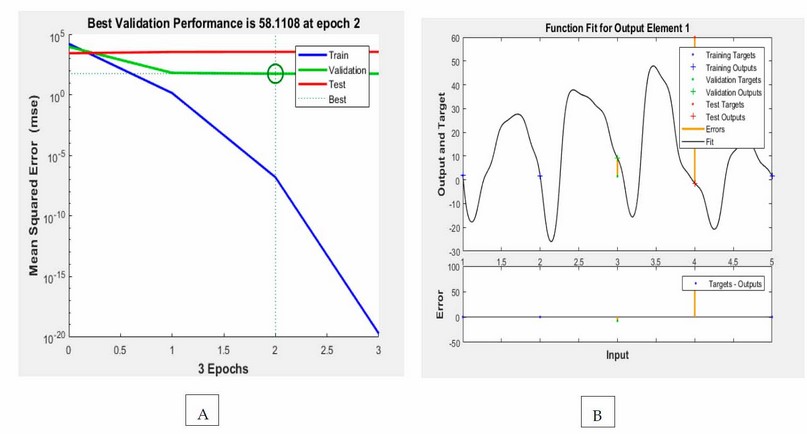
Figure 2. Diseases free. Neural network's performance between the input and the mean square of the regression,
CONCLUSIONS
Automated sorting by image analysis and the use of an artificial neural network showed high efficiency and accuracy compared to traditional sorting to fruit surface area, and recorded results were 1336.65, 1334.67 and 1332.06 cm2, respectively, with rates of 1334.46 cm2 and fruit circumference shape then color, and recorded results were 57.53 cm2. Therefore, automatic sorting, like free diseases, should be resorted to obtain good results. It shows the value of free conditions. The performance of the neural network between the input and the mean square of the regression, as it recorded the best value to validate the performance and it was 58.11 in the second period
Acknowledgments
The Authors thank the staff of the College of Science, Department of Computer Science at the University of Baghdad, and specifically the Image processing Laboratory, for their assistance in my work.
REFERENCES
1- Arjenaki, O. O., Moghaddam, P. A., & Motlagh, A. M. (2013). Online tomato sorting based on shape, maturity, size, and surface defects using machine vision. Turkish Journal of Agriculture and Forestry, 37(1), 62-68.
2- Banerjee, S. (2022). Automated Methodology for Volume Fraction Measurement of Three Phase Steel Micrograph Using Image Processing Techniques. Iraqi Journal of Science, 4601-4608.
3- Eduardo Carrillo and Alexander Aristizabal Penaloza, "Artificial vision to assure coffee-Excelso beans quality," in EATIS, Czech Republic, pp. 35,2009
4- Ewida H. Esmail,( 2015). Computer applications on pattren recognition for agricultural purposes. A thesis submitted in partial fulfillment of The requirements for the degree of doctor of philosophy. Ain Shams University
5- Fadel, F. H., & Behadili, S. F. (2022). A Comparative Study for Supervised Learning Algorithms to Analyze Sentiment Tweets. Iraqi Journal of Science, 2712-2724.
6- Hadi, S. M., Abdullah, M. M., & Mahdi, M. S. (2022). Computer Vision of Optimal Geometric Concentration Ratio for the Solar Ball Lens. Iraqi Journal of Science, 3727-3736.
7- Hongshe Dang, Jinguo Song, Qin Guo, "A Fruit Size Detecting and Grading System Based on Image Processing", 2010 Second International Conference on Intelligent Human-Machine Systems and Cybernetics, vol. 2, pp. 83-86, August 2010.
8- Hussein, N. A. K., & Al-Sarray, B. (2022). Deep Learning and Machine Learning via a Genetic Algorithm to Classify Breast Cancer DNA Data. Iraqi Journal of Science, 3153-3168.
9- Kline, D. E., Surak, C., & Araman, P. A. (2003). Automated hardwood lumber grading utilizing a multiple sensor machine vision technology. Computers and electronics in agriculture, 41(1-3), 139-155.
10- Lamprinopoulou, C., Renwick, A., Klerkx, L., Hermans, F., & Roep, D. (2014). Application of an integrated systemic framework for analysing agricultural innovation systems and informing innovation policies: Comparing the Dutch and Scottish agrifood sectors. Agricultural Systems, 129, 40-54. doi:10.1016/j.agsy.2014.05.001
11- M. Khojastehnazhand, M. Omid, and A. Tabatabaeefar, "Development of a lemon sorting system based on color and size," African Journal of Plant Science, vol. 4(4), pp. 122-127, April 2010.
12- Mohammed, Z. K., & Abdullah, N. A. (2022). Survey For Arabic Part of Speech Tagging based on Machine Learning. Iraqi Journal of Science, 2676-2685.
13- Oraibi, M. R., & Radhi, A. M. (2022). Enhancement Digital Forensic Approach for Inter-Frame Video Forgery Detection Using a Deep Learning Technique. Iraqi Journal of Science, 2686-2701.
14- P. Vimala Devi, K. Vijayarekha, "Machine Vision Applications to Locate Fruits, Detect Defects and Remove Noise: A Review" RASAYAN J. Chem. Vol. 7, 2014.
15- Pramod G. Devalatkar, Shilpa R. Koli, "Identification of Age Factor of Fruit (Tomato) using Matlab- Image Processing", International Journal of Recent Trends in Engineering & Research (IJRTER)Vol. 02, Issue. 07, pp. 7-13, July – 2016
16- Salman, Asmaa Khudhair,(2021). Assessment the Drought Tolerance Criteria of Some Tomato Genotypes and Detection the Expression WRKY1. Mahali. Doctor Of Philosophy In Horticulture and Landscape Gardening (Pomology). University Of Baghdad,Iraq.
17- Wang, H. H., and D. W. Sun (2002c). Melting characteristics of cheese: analysis of the effects of cheese dimensions using image processing techniques. J. of Food Eng., 52(3), 279–284.
18- Zhang Y, Yin X, Zou X, Zhao J (2009) On-line sorting maturity of cherry tomato by machine vision. IFIP AICT 295: 2223–2229.
Received: January 15, 2023 / Accepted: February 25, 2023 / Published:15 March 2023
Citation: M M. Aljuboury, Abbas Jebur H. Effect of traditional and automated sorting on some tomato's properties.
Revis Bionatura 2023;8 (1) 66. http://dx.doi.org/10.21931/RB/2023.08.01.66